A hip fracture can cause life-threatening complications. Accurate diagnosis is essential when evaluating the patient’s overall health, medical condition and treatment. Treatment for hip fractures typically involves a combination of surgery, rehabilitation and medication. Overall, this involves a collaborative effort between radiologists and orthopedists.
A team of radiologists and orthopedists from the Center for Intelligent Imaging, or ci2, at UCSF recently investigated the feasibility of automatic identification and classification of hip fractures using deep learning which has the potential to improve outcomes by reducing diagnostic errors and decreasing time to operation. Their research was recently published in Radiology: Artificial Intelligence as a cover story.
As part of the study, scientists reviewed hip and pelvic radiographs from 1118 studies and labelled 3026 hips via bounding boxes and classified them as normal, displaced femoral neck fracture, nondisplaced femoral neck fracture, intertrochanteric fracture, previous open reduction and internal fixation, or previous arthroplasty. A deep learning–based object detection model was trained to automate the placement of the bounding boxes. From there, a densely Connected Convolutional Neural Network (or DenseNet) was trained on a subset of the bounding box images. The performance of the deep learning model was compared with two groups of human observers: 1) fellowship-trained radiologists and orthopedists; 2) senior residents in emergency medicine, radiology and orthopedics.
Overall, the results showed that the binary accuracy for detecting a fracture of this model was 93.7% with 93.2% sensitivity and 94.2% specificity. When comparing results with human observers, the accuracy of the model achieved an expert-level classification. Also, when the model was used as an aid, it improved human performance; aided resident performance approximated unaided fellowship training performance. With these results, the team concluded that a deep learning model can identify and classify hip fractures with expert performance, at the very least, when used as an aid for human performance.
“The best outcome of this project was the demonstration that human/machine collaboration is possible, and it can improve the performance of trainees or less experienced radiologists,” says Valentina Pedoia, PhD, assistant professor in the UC San Francisco Department of Radiology and Biomedical Imaging and one of the authors of this research. “We want all our patients to have the best possible care, and hopefully this technology will allow for a more efficient diagnosis and improve triage and outcomes as the time to surgery is decreased.”
UCSF launched the ci2 in Fall 2019 to accelerate the application of artificial intelligence (AI) technology to radiology, leveraging advanced computational techniques and industry collaborations to improve patient diagnoses and care.
“Ci2 is working on implementing this work into the clinical work flow right now,” says Dr. Pedoia. “I am excited to see this technology make a real difference in patient management.”
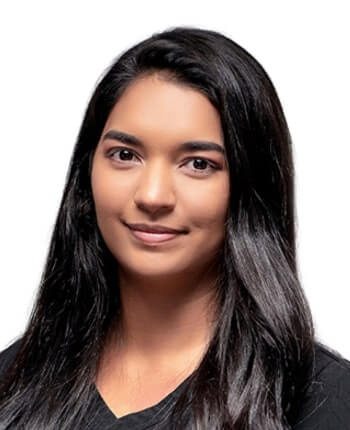